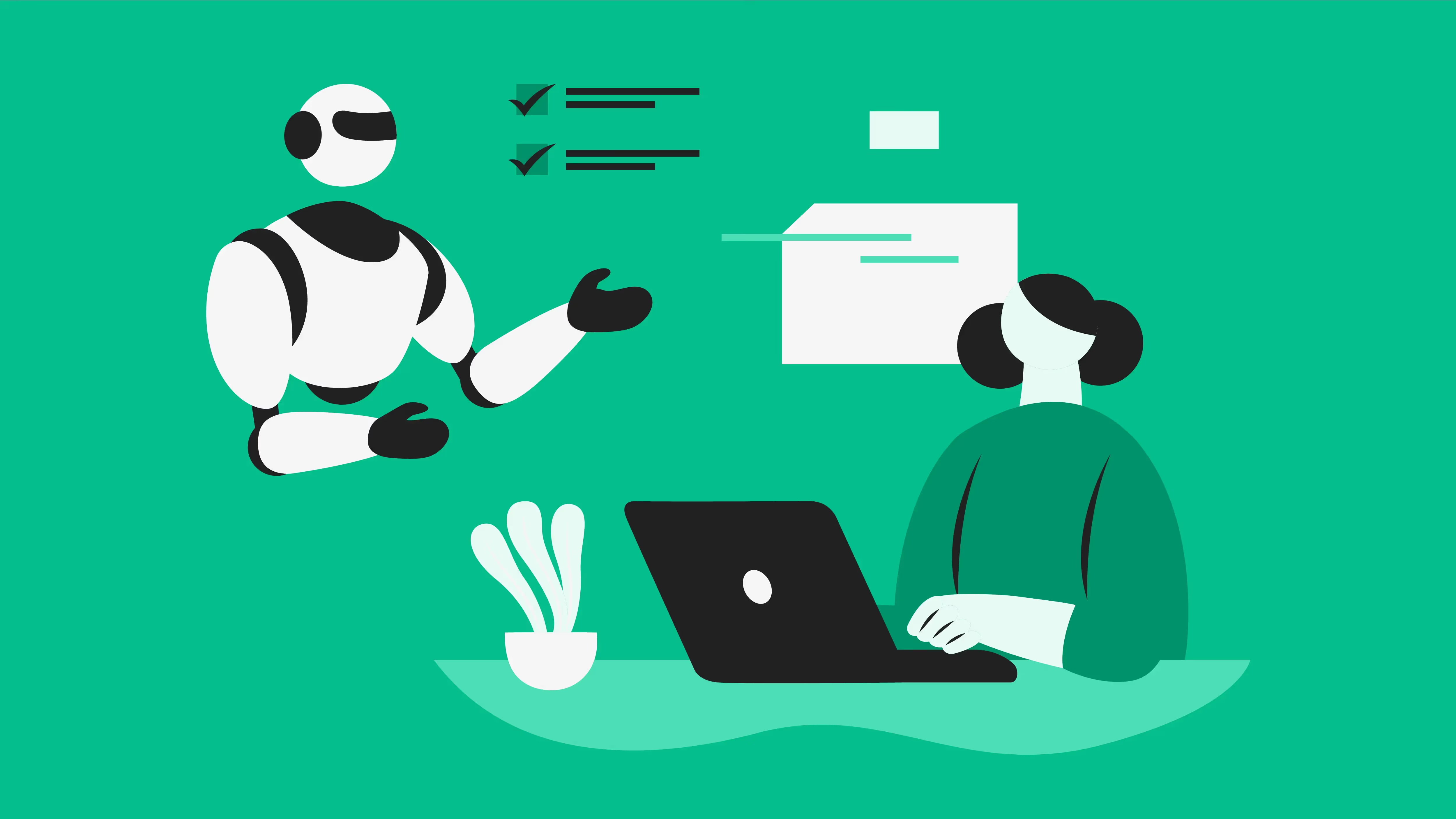
OpenAI vs Traditional NLP Models: A Comparative Analysis
The Rise of Advanced Language Models
In the rapidly evolving landscape of artificial intelligence (AI), natural language processing (NLP) has witnessed remarkable advancements in recent years. At the forefront of this revolution are groundbreaking language models developed by companies like OpenAI, which have transformed how machines understand, generate, and interact with human language.
OpenAI's Language Models
OpenAI, a renowned AI research company, has made significant strides in pushing the boundaries of what's possible in language AI. Their flagship models, such as GPT-3 and GPT-J, have demonstrated unprecedented abilities in text generation, question answering, and even code writing. These models leverage the power of deep learning to extract and manipulate complex linguistic patterns, enabling them to produce human-like text with remarkable coherence and fluency.
Traditional NLP Approaches
While OpenAI's models have captured widespread attention, the field of NLP has a long and rich history of traditional techniques and models. Established methods like rule-based systems, statistical models, and earlier neural network architectures have laid the foundation for modern advancements. These traditional approaches have their own strengths, such as interpretability, robustness, and specialized capabilities in domains like sentiment analysis and named entity recognition.
Comparative Analysis
Accuracy and Fluency
When it comes to accuracy and fluency in natural language tasks, OpenAI's models have demonstrated remarkable performance, often outpacing traditional NLP techniques. The sheer scale of data and computational power behind the training of GPT-3 and similar models has allowed them to capture intricate linguistic patterns and generate highly coherent and contextually appropriate text.
Interpretability and Generalization
However, traditional NLP approaches, such as rule-based systems and statistical models, often excel in areas requiring precise, interpretable, and domain-specific understanding. For example, traditional methods may be more robust in tasks like sentiment analysis or named entity recognition, where the ability to explain the reasoning behind the model's decisions is crucial.
%20(1).avif)
In terms of generalization capabilities, OpenAI's models have shown impressive versatility, adapting to a wide range of language-related tasks with limited fine-tuning. In contrast, traditional NLP models may be more specialized and require extensive retraining to handle novel domains or inputs.
Key Considerations
Throughout the analysis, we will consider factors such as:
- Accuracy and fluency in natural language tasks
- Generalization capabilities and robustness to diverse inputs
- Interpretability and transparency of the models
- Adaptability to specialized domains and use cases
- Computational efficiency and resource requirements
By understanding the trade-offs and complementary nature of these approaches, we can gain valuable insights into the evolving landscape of language AI and make informed decisions about the best tools and techniques to employ in various applications.
Conclusion
The competition and collaboration between OpenAI's advanced language models and traditional NLP techniques are shaping the future of artificial intelligence in the realm of natural language understanding and generation. By exploring the nuances of this dynamic landscape, we can unlock new possibilities for intelligent systems that seamlessly integrate human-like communication and reasoning capabilities.